With the boom of video doorbells with the likes of Ring, Skybell and Next doorbell cams, I came to the realization that I did not want to be cloud dependent for this type service for long term reliability, privacy and cost.
I finally found last year a wifi video doorbell which is cost effective and support RTSP and now ONVIF streaming:
The RCA HSDB2A which is made by Hikvision and has many clones (EZViz, Nelly, Laview). It has an unusual vertical aspect ratio designed to watch packages delivered on the floor....
It also runs on 5GHz wifi which is a huge advantage. I have tried running IPCams on 2.4GHz before and it is a complete disaster for your WIFI bandwidth. Using a spectrum analyzer, you will see what I mean. It completely saturates the wifi channels because of the very high IO requirements and is a horrible design. 2.4GHz gets range but is too limited in bandwidth to support any kind of video stream reliably... unless you have a dedicated SSID and channel available for it.
The video is recorded locally on my NVR. I was able to process the stream from it on Home Assistant to get it to do facial recognition and trigger automations on openLuup like any other IPCams. This requires quite a bit of CPU power to do...
I also get snapshots through push notifications through pushover on motion like all of my other IPcams. Movement detection is switched on and off by openLuup... based on house mode.
Sharing a few options for object recognition which then can be used as triggers for home automation.
My two favorites so far:

Object detection for video surveillance. Contribute to asmirnou/watsor development by creating an account on GitHub.

Automated CI toolchain to produce precompiled opencv-python, opencv-python-headless, opencv-contrib-python and opencv-contrib-python-headless packages. - GitHub - opencv/opencv-python: Automated CI...
I have optimized my facial recognition scheme and discovered a few things:
My wifi doorbell, the RCA HSDB2, was overloaded by having to provide too many concurrent rtsp streams which was causing the streams themselves to be unreliable:
Cloud stream
stream to QNAP NVR
stream to home assistant (regular)
stream to home assistant facial recognition.
I decided to use the proxy function of the QNAP NVR to now only pull 2 streams from the doorbell and have the NVR be the source for home assistant. This stabilized the system quite a bit.
The second optimization was to find out that by default home assistant processes images every 10s. It made me think that the processing was slow but it turns out that it was just not being triggered frequently enough. I turned it up to 2s and now I have a working automation to trigger an openLuup scene, triggering opening a doorlock with conditionals on house mode and geofence. Now I am looking to offload this processing from the cpu to an intel NCS2 stick so I might test some other components than Dlib to make things run even faster.
Can we? Simply? Specifically Foscams which used to run 'fine' on Vera but are not exactly high importance to me.
But since they are there....
Cheers
C
Sharing what I have learned and some modifications to components with their benefits.
On home assistant/python3, facial recognition involves the following steps:
Even though a few components have been created on home-assistant for many years to do this, I ran into challenges which forced me to improve/optimize the process.
Home Assistant's camera does not establish and keep open a stream in the background. It can open one on demand through its UI but doesn't keep it open. This forces the facial camera component to have to re-establish a new stream to get a single frame every time it needs to process an image causing up to 2s of delays, unacceptable for my application. I therefore rewrote the ffmpeg camera component to use opencv and maintain a stream within a python thread and since I have a GPU, I decided to decode the video using my GPU to relieve the CPU. This also required playing with some subtleties to avoid uselessly decoding frames we won't process while still needing to remove them from the thread buffer. The frame extraction was pretty challenging using ffmpeg which is why I opted to use opencv instead, as it executes the frame synchonization and alignment from the byte stream for us. The pre-set pictures was not a problem and a part of every face component. I started with the dlib component which had two models for ease of use. It makes use of the dlib library and the "facial_recognition" wrapper which has a python3 API but the CNN model requires a GPU and while it works well for me, turned out not to be the best as explained in this article and also quite resource intensive:https://www.learnopencv.com/face-detection-opencv-dlib-and-deep-learning-c-python/So I opted to move to the opencv DNN algorithm instead. Home Assistant has an openCV component but it is a bit generic and I couldn't figure out how to make it work. In any case, it did not have the steps 5 and 6 I wanted. For the face encoding step, I struggled quite a bit as it is quite directly connected to what option I would chose for step 6. From my investigation, I came to this: https://www.pyimagesearch.com/2018/09/24/opencv-face-recognition/
"*Use dlib’s embedding model (but not it’s k-NN for face recognition)
In my experience using both OpenCV’s face recognition model along with dlib’s face recognition model, I’ve found that dlib’s face embeddings are more discriminative, especially for smaller datasets.
Furthermore, I’ve found that dlib’s model is less dependent on:
Preprocessing such as face alignment
Using a more powerful machine learning model on top of extracted face embeddings
If you take a look at my original face recognition tutorial, you’ll notice that we utilized a simple k-NN algorithm for face recognition (with a small modification to throw out nearest neighbor votes whose distance was above a threshold).
The k-NN model worked extremely well, but as we know, more powerful machine learning models exist.
To improve accuracy further, you may want to use dlib’s embedding model, and then instead of applying k-NN, follow Step #2 from today’s post and train a more powerful classifier on the face embeddings.*"
The trouble from my research is that I can see some people have tried but I have not seen posted anywhere a solution to translating the location array output from the opencv dnn model into a dlib rect object format for dlib to encode. Well, I did just that...
For now I am sticking with the simple euclidian distance calculation and a distance threshold to determine the face match as it has been quite accurate for me but the option of going for a much more complex classification algorithm is open... when I get to it.So in summary, the outcome is modifications to:
A. the ffmpeg camera component to switch to opencv and enable background maintenance of a stream with one rewritten file:
https://github.com/rafale77/home-assistant/blob/dev/homeassistant/components/ffmpeg/camera.py
B. Changes to the dlib face recognition component to support the opencv face detection model:
https://github.com/rafale77/home-assistant/blob/dev/homeassistant/components/dlib_face_identify/image_processing.py
C. Modified face_recognition wrapper to do the same, enabling conversion between dlib and opencv
https://github.com/rafale77/face_recognition_models/blob/master/face_recognition_models init.py
https://github.com/rafale77/face_recognition_models/tree/master/face_recognition_models/models
Overall these changes significantly improved speed and decreased cpu and gpu utilization rate over any of the original dlib components.
At the moment the CUDA use for this inference is broken on openCV using the latest CUDA so I have not even switched on the GPU for facial detection yet (it worked fine using the dlib cnn model) but a fix may already have been posted so I will recompile openCV shortly...
Edit: Sure enough openCV is fixed. I am running the face detection on the GPU now.
At the moment i'm using the Surveillance software in Synology but I'm limited to 6 cameras (2 included and I took a 4pack a while ago)
But I have 8 cameras, so right now, 2 of them are not in the NVR!
I checked back in time motioneye but this software is very slow and all my cameras feed was lagging...
any other solution? 😉
Something fun to do if you have a camera located on your driveway:
This home assistant component enables recognition of a license plate which in turn could open the garage door...
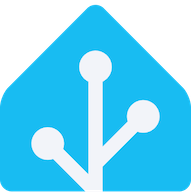
The integration you requested has been removed
Sharing an excellent skill I use to locally stream from my IPCams to echo shows:
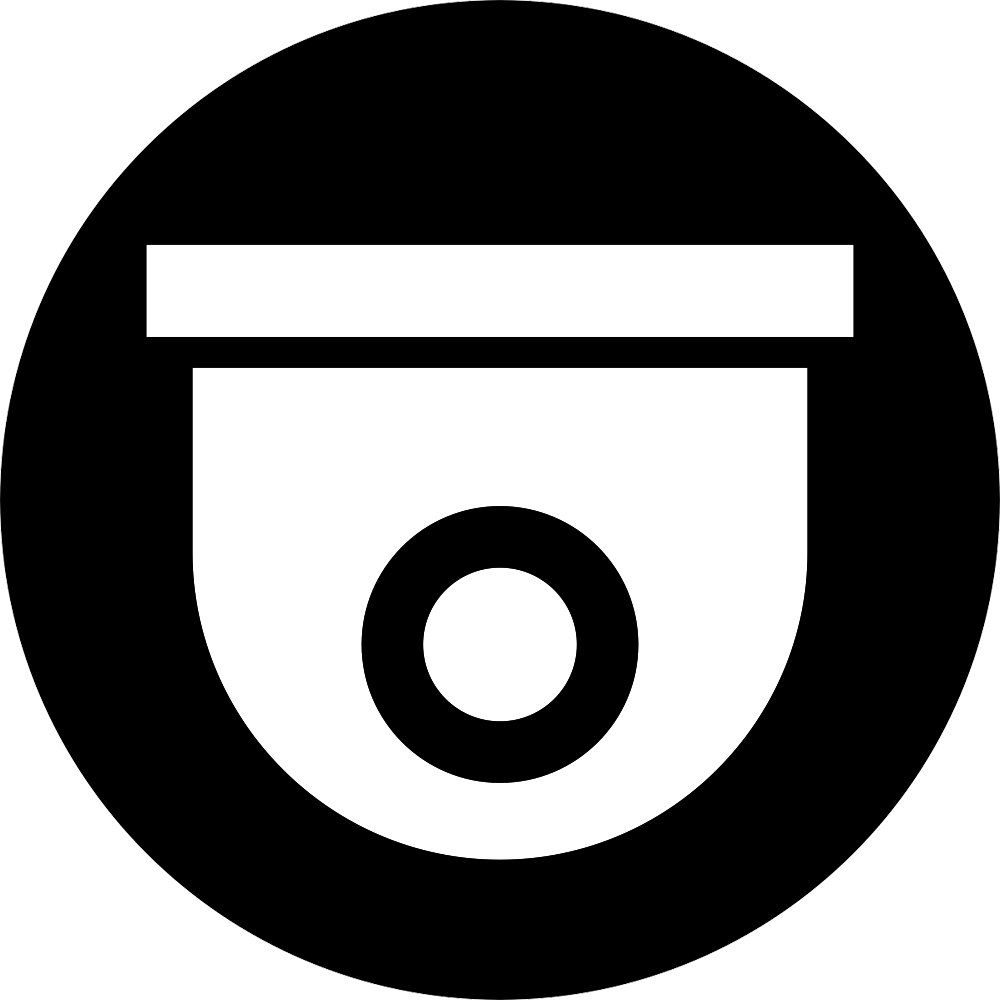
Your video stream does not need to go to the cloud. This skill just forwards the local stream address to the echo device when the camera name is called. It does require them to host the address and camera information (credentials) on their server though. I personally block all my IP cameras from accessing the internet from the router.
Facial recognition triggering automation
-
I have optimized my facial recognition scheme and discovered a few things:
My wifi doorbell, the RCA HSDB2, was overloaded by having to provide too many concurrent rtsp streams which was causing the streams themselves to be unreliable:
Cloud stream
stream to QNAP NVR
stream to home assistant (regular)
stream to home assistant facial recognition.I decided to use the proxy function of the QNAP NVR to now only pull 2 streams from the doorbell and have the NVR be the source for home assistant. This stabilized the system quite a bit.
The second optimization was to find out that by default home assistant processes images every 10s. It made me think that the processing was slow but it turns out that it was just not being triggered frequently enough. I turned it up to 2s and now I have a working automation to trigger an openLuup scene, triggering opening a doorlock with conditionals on house mode and geofence. Now I am looking to offload this processing from the cpu to an intel NCS2 stick so I might test some other components than Dlib to make things run even faster.
-
This is what I am looking at implementing at the moment:
It is an API which could potentially work with an openLuup plugin.
-
Well after much consideration, I decided to add an nVidia GPU to my NAS which is already hosting all my automation VMs to support this instead of getting a dedicated SBC with a coprocessor. It seems to be the simplest and most cost effective way while probably offering the best performance and extendibility for future AI.
-
Your hardware will never cease to amaze me! I bet one of your tesla cards costs more than my entire setup which is already not what one would call puny... COVID19 seems to have jacked up all the prices and made availability of hardware quite limited. I am seeing the GPUs I was shopping all going out of stock or raising prices within a couple of days...
Back on the topic, my wife was pretty happy with the door unlocking within 3s of us showing up in front of the doorbell. I can sense a delay for the integration to pickup the video stream, resolve it and run the facial recognition which is why I am looking at testing it with a GPU which may open the door to do other things like recognizing when a package was delivered and eventually filter out camera motion signals to only show when there is an actual person. I am even thinking about detecting deers in the yard to start my sprinkler to scare them away when they come to eat my flowers...
-
So I have not yet received my GPU but discovered that most of the reliability issues I am having is around getting a snapshot from my doorbell with ffmpeg. It seems to fail to get a picture to process between 15 and 30% of the time and I can't figure out why as I see no error from the proxy streamer. It does take ~2-10s for a full process using a single thread from my CPU at the moment and the CPU does not even appear to be overloaded during processing, hitting 12% load. hope the GPU will fix this.
-
Further info on this. Because my doorbell cam stream resolution is pretty high (1536x2048), and I am running all the automation within virtual machines, the iGPU of the CPU is not getting passed through so there is no hardware acceleration for the video stream decoding. This is why I am having dropped frames. I got the thing to work very reliably now, it is just a bit too slow to my taste (2-10s). I really need a GPU to passthrough to the VM for this and have it accelerate the video decoding and the deep learning facial recognition. Decided to cancel my unshipped 1650 super and got myself a 2060 super instead which has neural processors... It also mean that I will have to wait even longer...
-
Been coding some python to modify Home Assistant components to use the GPU both for video stream decoding and the API calls for recognition inferences. Speed is much improved and CPU/RAM load massively reduced. Pretty amazing how much memory one inference uses. My GPU has 8GB and uses 3GB to process one video stream with dlib... The inference time has gone from 2s down to near instant now. Most of the lag if any is to capture the screenshot to process.
-
A quick writeup on what I did on my VM:
- Powered off my NAS and inserted in the GPU. Power on the NAS and setup the GPU to passthrough into the VM from the VM supervisor (In my case QNAP Virtual Station)
- Install the Geforce driver. https://www.nvidia.com/Download/index.aspx?lang=en-us
- Install Cuda Toolkit https://docs.nvidia.com/cuda/cuda-installation-guide-linux/index.html
- Install cuDNN (downloading the library requires registering for a dev account with nVidia)
- Compile ffmpeg to support GPU and install it: https://developer.nvidia.com/ffmpeg
- Compile dlib to support CUDA: https://stackoverflow.com/questions/49731346/compile-dlib-with-cuda and install it
- Changed my home assistant camera ffmpeg command to add the nvidia header as documented by the ffmpeg documentation above.
- Modified Home Assistant's dlib component to activate cnn model. This is the one file I changed: https://github.com/rafale77/home-assistant/blob/dev/homeassistant/components/dlib_face_identify/image_processing.py
Not going to detail the home assistant configuration changes here since they are very installation dependent. Also I did this on linux but it is very platform agnostic...
9. Create an openLuup virtual device (can be a motion or door sensor, in my case I used a virtual switch)
10. Bind the Home Assistant entity to that created device using home assistant automation and an API calls updating switch status and another variable capturing the name of the person recognized.
11. Created a scene with the code below to resolve what face was recognized and unlock the door if house mode just changed to home and nobody has yet entered the house, a condition I isolate using a global variable in openLuup (greet):local Fid = **virtual switch id** local lockid = **id of the lock** local SS_SID = "urn:micasaverde-com:serviceId:SecuritySensor1" local VS_SID = "urn:upnp-org:serviceId:VSwitch1" local face = luup.variable_get(VS_SID,"Text2",Fid) local last = luup.variable_get(SS_SID, "LastTrip",Fid) if string.find(face, "hubby") ~= nil then face = string.format("Hubby @ %s", os.date()) luup.variable_set(SS_SID, "LastFace", face,Fid) if greet == 1 then luup.variable_set(SS_SID, "LastTrip", os.time(),Fid) luup.call_action("urn:micasaverde-com:serviceId:DoorLock1","SetTarget",{newTargetValue = 0}, lockid) sendnotifcam("doorbell") end elseif string.find(face, "wify") ~= nil then face = string.format("wify @ %s", os.date()) luup.variable_set(SS_SID, "LastFace", face,Fid) if greet == 1 then luup.variable_set(SS_SID, "LastTrip", os.time(),Fid) luup.call_action("urn:micasaverde-com:serviceId:DoorLock1","SetTarget",{newTargetValue = 0}, lockid) sendnotifcam("doorbell2") end else return false end
Note that I also have a global function to send a push notification with snapshots in my startup lua. (sendnotifcam)
This is the peak load when the GPU is resolving the face from my doorbell, which I set to once per second:
-
because of my choice of driver/cuda and cudnn version... I am on the bleeding edge and am having to compile everything from source... opencv is a bit of a nightmare with me having to change some of the source code but some other libraries are also missing. I am currently doing caffe/pytorch... after completing ffmpeg and dlib... All good fun and learning a lot about deep learning but it is pretty time consuming.
-
Alright!!! I got one thing fixed on home assistant's camera components:
So the issue is that all these image processing component work by grabbing a snapshot from a camera component.
The issue with all these camera components is that they actually don't stream in the background in home assistant and therefore every time one needs to get a snapshot, a new connection needs to be negotiated and established. This process, uses ffmpeg and the time it takes to establish this connection varies a lot even for one same camera. It can also fail for various reasons.
Now this has cause my facial recognition to have latency varying by a lot from instant to near 10s in my extensive testing. Grabbing the snapshot itself only takes 0.03s and processing it now with my GPU also only takes 0.1s... I have been chasing this latency problem and have even gone as far as learning enough Python coding to try rewrite the ffmpeg camera component using threading. Then I discovered this wrapper: https://github.com/statueofmike/rtspAnd have now integrated it into the ffmpeg component. Boom! Home assistant now constantly stream from the camera (technically a proxy which is already on the same machine) and never has any lag. I also took advantage of this to fix a few bugs in the amcrest camera component... which I will be posting on my fork of home assistant.
One more problem resolved!
Now opencv, tensorflow and pytorch are not supporting the latest nvidia cuda and cudnn versions yet so I will sit back on these and use dlib which I also modified to make use of the GPU.
My mods for reference:
Next step: Object detection! let's try people and packages... this would eliminate false motion alarms from cameras when we get a windstorm here.
-
I have not been posting in this thread for some time but it is an area I have been spending my spare time on... learning about this area of active research....
From the previous component I rewrote, I have now evolved my face recognition component with different models. Here a summary table bellow:
With some interesting reads:
-
I have taken another look at this and have now drastically modified my fork of homeassistant and deviated from the main branch:
- I added a state variable to the image processing component so as to turn on and off the processing from openLuup. (and giving the ability to turn on only upon camera movement detection.)
- Massively optimized the video frame handling so that it doesn't get converted into a variety of formats. It's still not perfect since it still goes from the GPU decoder to the CPU, runs some processing (resizing mostly) and gets sent back to the GPU for model inference but I will get there eventually.
- Huge improvement in the face detection model where I refactored the model to take out some constants, preventing them from getting recalculated for every frame and more than doubled the inference speed.
- Updated my object detection model to this improved version of Yolo which is more accurate and only cost a tiny bit more:
Note: I am heavily relying on pytorch as my neural network framework (supported by facebook but initiated as a lua project before it moved to python) and opencv for video and image processing (supported by intel).